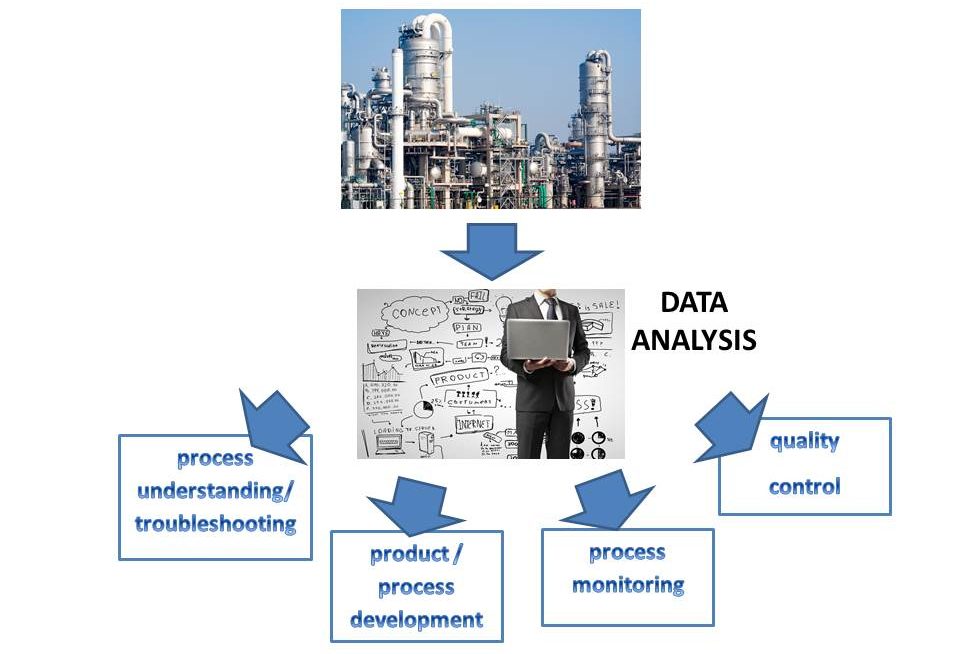
Current sensor technology (ranging from simple flow meters to process analyzers to near infra-red spectrometers to digital cameras) makes process measurements available at a much faster rate and a much lower cost than just a few years ago. Consequently, massive amount of data (“big data“) coming from manufacturing processes are now routinely available in real time to the process engineers. This has led to a widespread diffusion of the use of data-driven models. Among these, models based on multivariate statistical techniques have demonstrated their great potential to exploit data (whether real-time or historical) in order to provide information about the process behavior and the product quality.
CAPE-Lab studies how to efficiently exploit this wealth of knowledge to assist several engineering activities of paramount importance, such as product and process design, process understanding and troubleshooting, process monitoring, product quality monitoring, and process control. In particular, statistical process control techniques allow one to improve the knowledge of the critical phases of the process, in such a way as to promptly detect process faults, to diagnose the cause of a fault, and to monitor the product quality in real time.
Data-driven models have proved an efficient tool to support the design of new products, and the transfer of products, processes and the respective technologies between scales, plants or production sites. Effective product and process transfer can ensure competitive advantages in terms of time and materials use savings, and can accelerate the time-to-market of new products.
Finally, multivariate statistical techniques are reliable methodologies for the development of innovative Process Analytical Technologies (PAT) and for the implementation of Quality-by-Design paradigms into pharmaceutical development and manufacturing activities.